In today’s digital landscape, unstructured data comprises a significant chunk of business data, approximately 90%, and it comes in unconventional forms like scanned copies, handwritten notes, emails, documents, images, audio and video forms, etc. It is safe to say anything you can’t fit into rows and columns falls under unstructured data. Besides, you cannot align them into predefined templates, making data processing a never-ending headache. Considering the intricacies of unstructured data, you might require a more advanced approach that prioritizes a context-aware approach rather than a superficial approach.
Challenges aside, why are businesses focusing on unstructured data?
Unstructured data is more than a challenge; it’s an opportunity. In today’s data-driven world, companies are trying to optimize business operations by making the most of their confidential data. Needless to say, all the intricate real-world data, such as regulatory filings, financial statements, and business reports, with untapped potential for insights, are predominantly unstructured.
Tapping into this information can help you unlock more profound operational insights, identify underlying trends or patterns in critical business data, and, most notably, fuel advanced technologies like Generative AI (GenAI) to unlock innovation and business automation.
Business leaders increasingly acknowledge that harnessing unstructured data like operational records and compliance documents can make them adjust to market fluctuations quickly, refine their data strategies, and drive better decision-making. It is time to address the elephant in the room: unstructured data processing is no longer prolonged chaos but more of a strategic initiative for innovation.
GenAI elevates IDP, streamlining unstructured data processing.
GenAI catalyzes intelligent document processing (IDP), transforming disorganized data into actionable insights. Moreover, exposing GenAI to raw, multiformat data can equip them with a better understanding of contexts, themes, and nuances. This increased exposure leads to the discovery of new use cases because GenAI can now connect the dots better, generate context-aware responses faster, and provide more relevant solutions.
In the bigger picture, incorporating GenAI in document processing has improved processing speed, paving the way for streamlined document processing at a large scale. While GenAI amplifies intelligent document automation, it has some setbacks that are hard to ignore. The most popular one is that LLMs tend to hallucinate in ambiguous situations, meaning they fabricate responses that may not be factually verified, creating an error-prone condition.
Another significant issue is that GenAI is heavily dependent on pre-trained data. It cannot access anything other than its training data, which can take a serious toll on its knowledge spectrum and cause it to produce less specific responses. GenAI can indeed support contextual intelligence, but the question is, to what extent? Unfortunately, notable discrepancies were reported when GenAI was left to process large, fragmented datasets.
Bridging gaps with retrieval augmented generation
Hindered by the limitations of AI document intelligence, businesses are now shifting focus to a retrieval augmented generation( RAG) based approach. Let us explore how RAG overcomes these barriers and propels IDP to the next level.
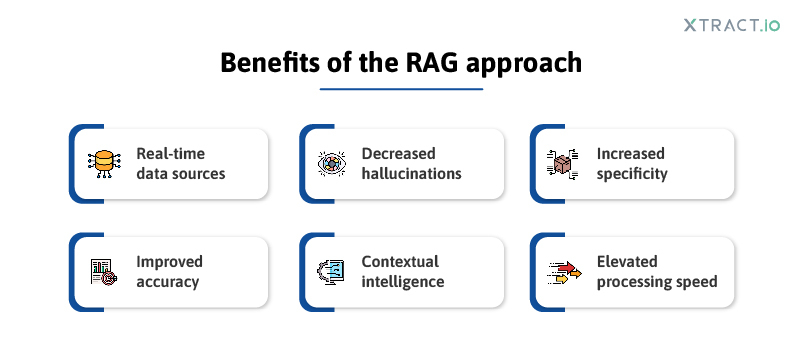
Firstly, RAG connects LLMs with external data sources like data repositories and vector databases containing proprietary data, company-specific internal documents, and industry-specific data. Feeding LLMs with domain-specific data can bring them one step ahead to generating context-rich responses and upgrade their performance with proprietary data extraction while navigating intricate business documents.
Moreover, connecting LLMs to real-time trusted data sources encourages responses that are grounded in accuracy, enabling confident and transparent decision-making processes.
Another interesting aspect is that every time RAG encounters a new case, it updates its memory through a feedback mechanism exercising its adaptive learning capabilities. This way, RAG has proved impactful even with exceptional cases, cutting down training periods and eliminating the need for custom training.
Choosing to embrace retrieval-augmented generation is one thing, but the most important thing is building RAG architecture.
Setting up a strong RAG infrastructure
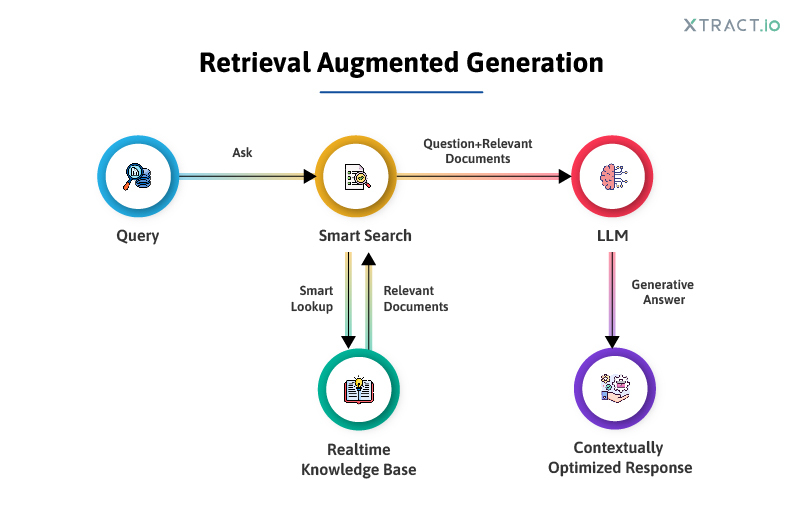
A RAG architecture consists of intricate processes: data ingestion, chunking, and embedding. Quality data is essential to enjoying RAG’s maximum potential. Attaining quality data starts with data ingestion, which involves supercharged data extraction, cleaning, and enrichment practices.
Post-data ingestion is the most critical chunking process, breaking down enormous datasets into manageable smaller chunks for better data retrieval. Chunking can be based on characters, pages, adaptive, and semantic. Choosing which type of chunking is based on factors such as the data’s nature, query length, and the complexity of user queries. Interestingly, the intended use of the retrieved data, whether for summarization, extracting insights, or other purposes, can significantly impact the selection process.
Then, embeddings convert the captured data to numerical format, making it LLM-readable content. Once embeddings are done, they must be stored in a vector database, indexed based on standard criteria for quicker data spotting and retrieval of the most relevant data, which powers GenAI’s generative process. Indexed data can increase overall operational efficiency by facilitating LLMs’ access to the most pertinent data in the least possible time without compromising data accuracy.
Leveraging RAG for intelligent document automation can be a perfect choice, but building it in traditional ways can be expensive and time-consuming. Most importantly, it takes specialized coding expertise to build RAG architecture, limiting business users from leveraging RAG.
RAG made easy with no-code
Worry no more. With recent advancements, no-code RAG employs an easy drag-and-drop interface, allowing business users to build RAG pipelines with zero coding knowledge. This reduces development time and effort and eliminates complexity. Using no-code RAG can superpower AI document processing efficiency rather than getting stuck with the intricate details of building RAG architecture from scratch.
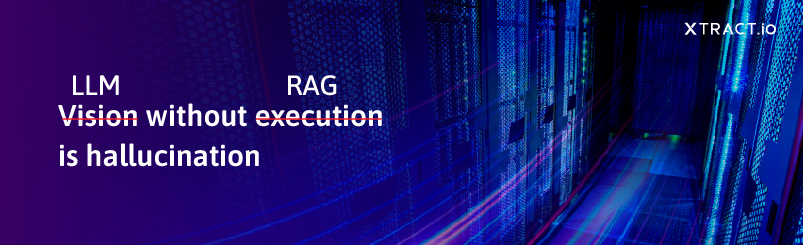
If you’re looking for a similar solution, XDAS offers advanced no-code RAG features to deliver enhanced contextual intelligence with access to public data sources. Book a demo with us today!
Wrapping up
The involvement of GenAI, most notably RAG, has transformed how businesses approach unstructured document processing. Businesses have realized that the scope of RAG in IDP is far more than increasing efficiency; it is more about innovating for better opportunities. Despite the intricate complexities, integration with RAG and specialized LLMs has helped businesses overcome hallucinations, privacy concerns, and security breaches. The future of AI document processing does not end here; it keeps evolving. As a first step, we should embrace the synergy of GenAI and RAG in intelligent document processing.